Imaging Seminar: Generalized locality for lightweight, robust AI-driven imaging
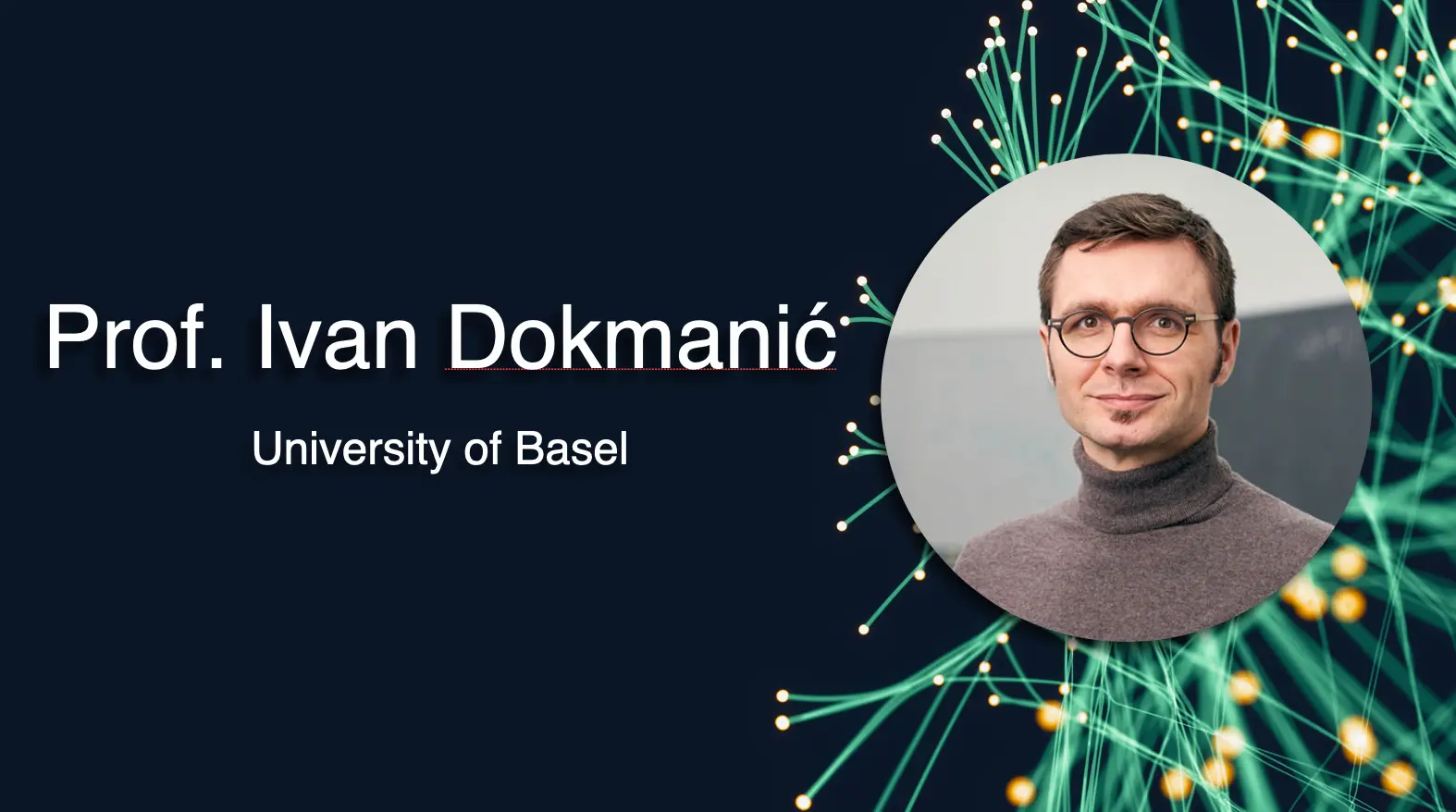
The seminar is jointly organised with IEM.
It will be followed by a lunch.
Abstract:
Deep learning enables imaging with less data, worse signal-to-noise ratio, and at higher speed and resolution than any prior technology. Yet, while its impact on the "downstream pipeline"—image enhancement, denoising, segmentation, interpretation, ...—has clearly been transformative, its full potential in recovering images from raw data in a way that fully leverages both physics and data remains, I believe, under-realized. Important imaging-driven scientific and medical applications are still often driven by traditional reconstruction, partly because deep nets are biased by training data and prone to hallucination. Compounding this are convenience assumptions about data and physics that don’t always align with the messy reality. In this talk, I’ll offer a subjective view of where deep learning stands in image reconstruction and outline ways to navigate some of the challenges. Drawing on work from my collaborators and myself, I’ll discuss how to build and train deep nets with desirable inductive biases which adapt to incomplete information about the forward models. We’ll explore how "localized" networks can act as effective priors, and how generalizing the notion of locality to the transform domain leads to lightweight deep reconstructors resilient to distribution shifts. These ideas impact both applications—notably memory-limited 3D imaging, including cryo-ET— and theory, through exciting connections with geometric machine learning and microlocal analysis.
Bio:
Ivan Dokmanić is an Associate Professor in the Department of Mathematics and Computer Science at the University of Basel, Switzerland. From 2016 to 2019 he was an Assistant Professor in the Coordinated Science Laboratory at the University of Illinois at Urbana-Champaign, where he now holds an adjunct appointment. He received a diploma in electrical engineering from the University of Zagreb in 2007, a PhD in computer science from EPFL in 2015, and did a postdoc at Institut Langevin and Ecole Normale Supérieure in Paris between 2015 and 2016. Before that he was a teaching assistant at the University of Zagreb, a codec developer for MainConcept AG, Aachen, and a digital audio effects designer for Little Endian Ltd., Zagreb. His research interests lie between inverse problems, machine learning, and signal processing. He received the Best Student Paper Award at ICASSP 2011, a Google PhD Fellowship, an EPFL Outstanding Doctoral Thesis Award and a Google Faculty Research Award. In 2019 the European Research Council (ERC) awarded him a Starting Grant.